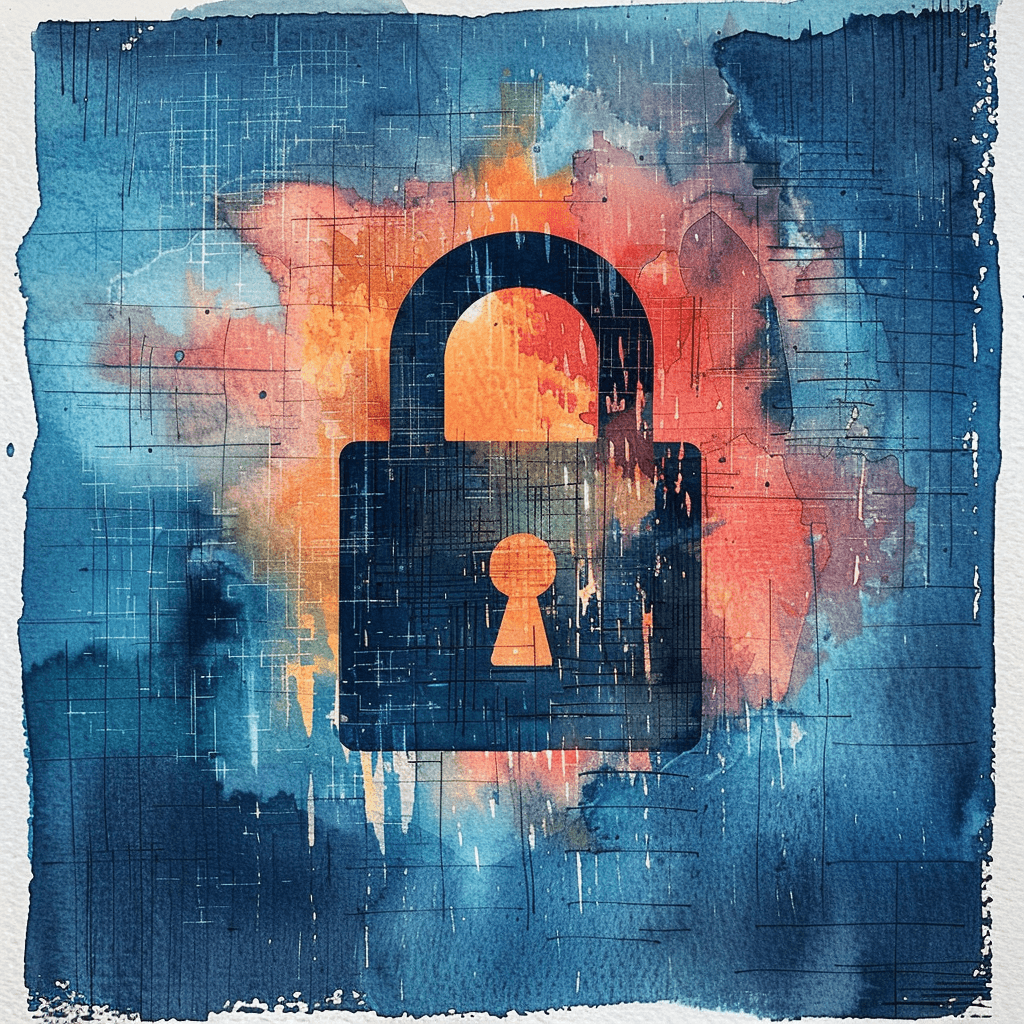
In an increasingly interconnected digital landscape, cybersecurity threats have evolved from occasional nuisances to persistent, sophisticated challenges that threaten business continuity and data integrity. At Codegig, we’re witnessing a paradigm shift as artificial intelligence revolutionizes how organizations detect, analyze, and respond to these evolving threats.
The Changing Threat Landscape
Today’s cybersecurity challenges bear little resemblance to those of even a few years ago:
- Attack sophistication has increased exponentially
- Attack surfaces have expanded with cloud adoption and remote work
- Threat actors now include nation-states and highly organized criminal enterprises
- Attack velocity has accelerated, with some threats spreading globally in minutes
These changes have rendered traditional, signature-based security approaches increasingly inadequate. In this environment, AI has emerged not just as an enhancement but as a necessity.
How AI is Transforming Cybersecurity Detection
Artificial intelligence is revolutionizing threat detection across multiple dimensions:
Pattern Recognition Beyond Human Capability
Modern AI security systems can:
- Analyze billions of events to identify subtle anomaly patterns
- Recognize attack signatures that evolve over time
- Detect deviations from baseline behavior across complex networks
- Identify correlations between seemingly unrelated events
This capability enables the detection of zero-day threats and sophisticated attacks that would evade traditional security measures.
Predictive Threat Intelligence
AI has transformed threat intelligence from reactive to predictive:
- Analyzing global threat data to forecast emerging attack vectors
- Identifying vulnerable system components before attackers exploit them
- Predicting potential attack paths through networks
- Monitoring dark web activities to anticipate new exploit development
Organizations leveraging predictive threat intelligence can prioritize security resources more effectively and address vulnerabilities before they’re exploited.
Behavioral Analysis and User Entity Behavior Analytics (UEBA)
Perhaps AI’s most powerful contribution is its ability to understand normal behavior and identify deviations:
- Creating baseline profiles for users, devices, and network segments
- Detecting unusual access patterns or data movements
- Identifying compromised credentials through behavior anomalies
- Recognizing insider threats through subtle behavior changes
These capabilities enable security teams to focus on genuine threats rather than false positives, dramatically improving efficiency.
Real-World Applications of AI in Cybersecurity
AI is creating transformative capabilities across the security landscape:
Malware Detection and Analysis
Traditional antivirus solutions relied on known signatures, leaving systems vulnerable to new threats. AI-powered systems now:
- Detect malware based on behavior rather than signatures
- Identify polymorphic malware that changes its code to evade detection
- Analyze code structure to recognize malicious intent
- Automatically generate protection against new variants
Organizations implementing these solutions report up to 95% reduction in successful malware attacks.
Network Traffic Analysis
AI has revolutionized how security teams monitor network activity:
- Real-time analysis of encrypted traffic without decryption
- Identification of command-and-control communications
- Detection of data exfiltration attempts
- Recognition of network reconnaissance activities
These capabilities provide early warning of attacks in progress, often before actual data breaches occur.
Automated Incident Response
AI is transforming how organizations respond to security incidents:
- Automated containment of compromised systems
- Dynamic adjustment of security controls in response to threats
- Prioritization of alerts based on risk assessment
- Orchestration of complex response workflows
Security teams leveraging these capabilities report 60% faster mean time to resolution for security incidents.
Threat Hunting
AI has enabled a shift from reactive to proactive security through enhanced threat hunting:
- Identifying subtle indicators of compromise
- Analyzing historical data for previously undetected breaches
- Tracking lateral movement within networks
- Revealing connections between disparate security events
The Advantages of AI-Enhanced Cybersecurity
The business benefits of AI integration extend far beyond technical security improvements:
Reduced Alert Fatigue and False Positives
Traditional security tools generate overwhelming volumes of alerts, many of them false positives. AI dramatically improves signal-to-noise ratios:
- Correlating multiple indicators to confirm genuine threats
- Learning from feedback to reduce false positive rates
- Prioritizing alerts based on risk assessment
- Providing context for faster human analysis
Security teams report 70-80% reductions in false positives after implementing AI-enhanced detection systems.
Addressing the Skills Gap
The cybersecurity industry faces a critical shortage of skilled professionals. AI helps organizations maximize the effectiveness of existing teams:
- Automating routine analysis and triage
- Providing decision support for complex scenarios
- Accelerating investigation through automated evidence gathering
- Enabling tier-1 analysts to handle more sophisticated threats
This leverage effect allows organizations to maintain robust security despite talent shortages.
Improved Compliance and Reporting
AI enhances regulatory compliance through:
- Continuous monitoring of compliance-related controls
- Automated documentation of security incidents and responses
- Risk quantification based on comprehensive data analysis
- Evidence gathering for audit purposes
These capabilities reduce the burden of compliance while improving the quality of documentation.
Implementation Considerations: Lessons from the Field
At Codegig, we’ve learned several key lessons about successful AI integration in cybersecurity:
Human-AI Collaboration is Essential
The most effective security operations leverage both human and artificial intelligence:
- AI handles volume, pattern recognition, and initial analysis
- Human analysts provide context, strategic direction, and final judgment
- Regular feedback loops improve AI performance
- Clear escalation paths ensure appropriate human involvement
This collaborative approach yields significantly better results than either AI or human analysis alone.
Data Quality Determines AI Effectiveness
AI security tools are only as good as the data they analyze:
- Comprehensive logging and monitoring are prerequisites
- Data normalization improves analysis quality
- Historical data enables better baseline establishment
- Regular data quality assessment is essential
We help clients establish robust data foundations before implementing AI security tools.
Integration with Existing Security Infrastructure is Critical
AI security tools must work seamlessly with existing security ecosystems:
- Integration with SIEM systems for comprehensive visibility
- Connection to threat intelligence platforms for context
- Interoperability with response orchestration tools
- Compatibility with governance and reporting frameworks
Our implementation approach emphasizes integration to maximize value from existing security investments.
Emerging Trends in AI Cybersecurity
Several key trends are shaping the future of AI in